SWE-Lancer: Can AI make it as a freelance software engineer?
OpenAI’s new benchmark puts major LLMs to the test on freelance software gigs.
In the ongoing debate about AI’s role in the workforce, one big question looms: can AI models actually replace human workers in skilled jobs?
To try and answer that question, researchers at OpenAI have developed a new benchmark, SWE-Lancer, to test if LLMs can complete real-world freelance software engineering tasks.
To build the benchmark, the researchers took 1,488 real software engineering jobs from Upwork, ranging from $50 bug fixes to $32,000 feature implementations. The tasks can be divided into two distinct categories:
Coding tasks — where models must generate working solutions for software issues.
Technical decision-making tasks — where models act as software engineering managers, choosing the best proposal from multiple freelancers.
The tasks were collectively valued at $1 million USD. This means that SWE-Lancer acts as a monetary benchmark for AI's capabilities. It doesn’t just measure the technical accuracy of LLMs but also the economic viability.
The results?
The best-performing model, Claude 3.5 Sonnet, earned just over $400,000 out of a possible $1 million. That means LLMs are already capable of handling a significant portion of software engineering tasks—though the most complex work still requires human expertise.
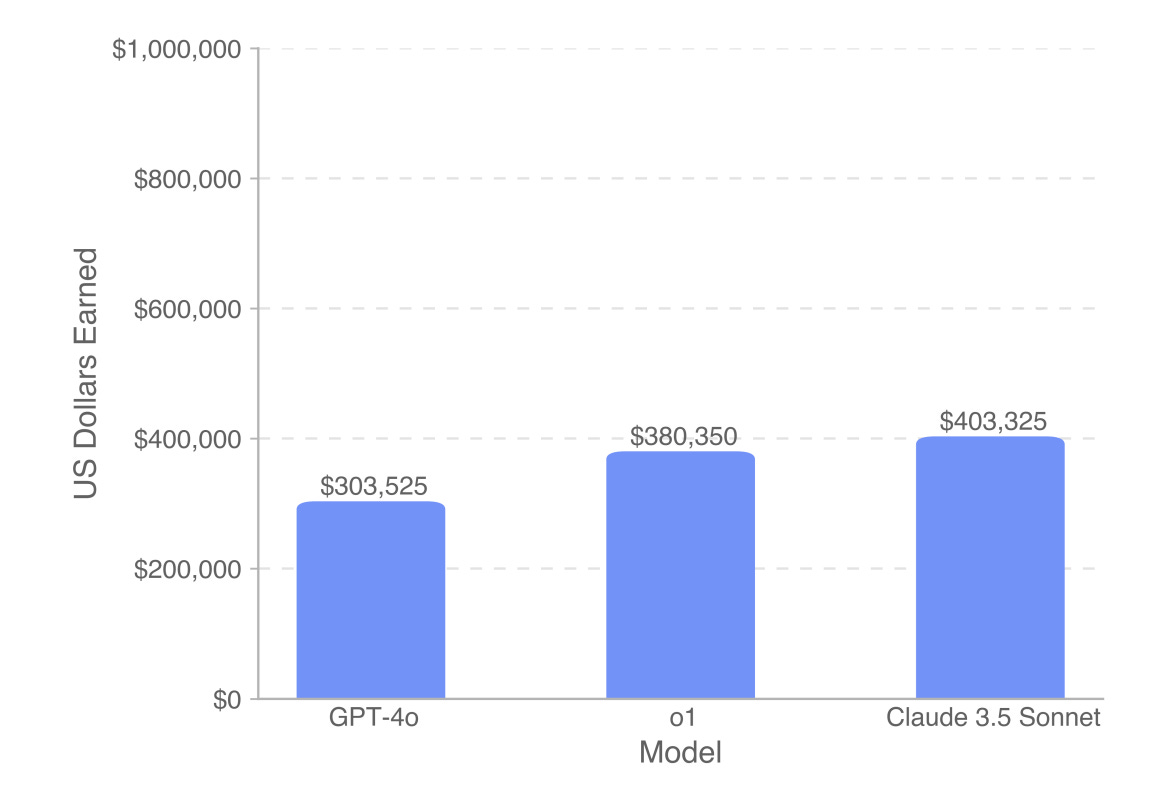
What is SWE-Lancer? A benchmark based on real-world work
Most AI coding benchmarks focus on small, self-contained tasks—solving algorithmic problems, completing isolated code snippets etc.
But real software engineering takes more than just writing a few lines of code. It’s about working across entire codebases, debugging complex issues, and integrating solutions into existing systems.
Instead of theoretical problems, SWE-Lancer pulls directly from freelance engineering work on Upwork. This means that every task in the dataset was originally completed by a human engineer for real payment.
This approach makes it the most realistic benchmark to date for evaluating AI’s potential as a software developer.
SWE-Lancer’s dataset
As mentioned earlier, the dataset for SWE-Lander is split into two main categories:
Individual Contributor (IC) software engineering tasks
These require the AI to generate working code solutions for bugs and feature requests.
Solutions are tested using end-to-end (E2E) tests, which simulate how a real user would interact with the software.
The grading process is triple-verified by human engineers to ensure accuracy.
SWE manager tasks
Here, the AI plays the role of a technical manager, evaluating multiple implementation proposals and choosing the best one.
Its choices are assessed against the actual decisions made by human engineering managers when the job was originally posted.
By testing both hands-on coding ability and higher-level decision-making, SWE-Lancer comprehensively assesses whether AI can function in a real engineering role.
So, can it?
How AI performed in the challenge
OpenAI’s researchers tested several frontier LLMs, including:
GPT-4o (OpenAI)
Claude 3.5 Sonnet (Anthropic)
o1 (OpenAI, high reasoning effort version)
This is how the models performed when evaluated on the full SWE-Lancer dataset ($1M total available earnings):
Claude 3.5 Sonnet earned the most, with $403K (40.3% of total earnings).
o1 followed with $380K (38.0%).
GPT-4o earned $304K (30.4%), placing last among the three.
Across all models, decision-making tasks (SWE Manager) had much higher accuracy rates than coding tasks (IC SWE). Even the best model, Claude 3.5 Sonnet, failed the majority of coding tasks, reinforcing that LLMs struggle with full-scale software engineering workflows.
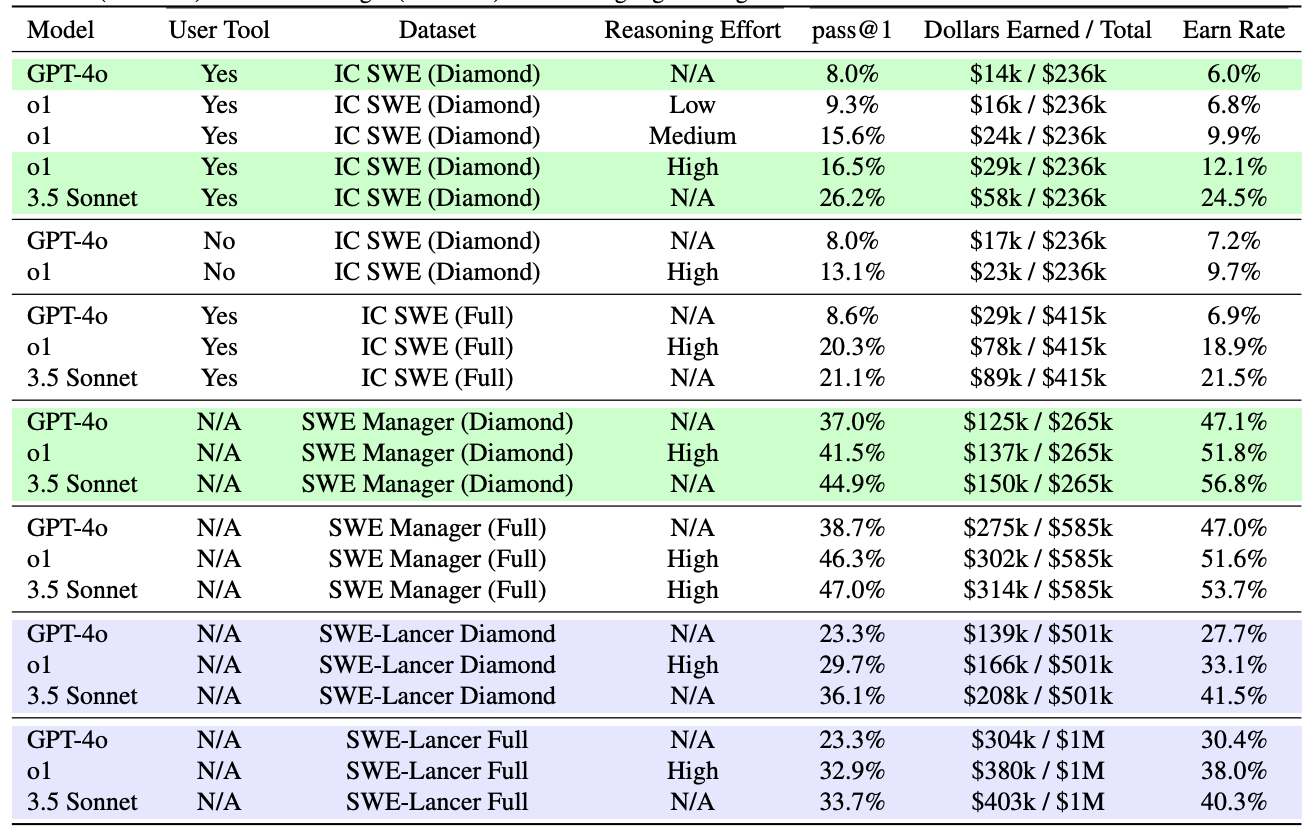
Performance breakdown by task type
Looking deeper into the data, AI models had varying success depending on the type of software engineering task.
Here’s an overview of where LLMs performed well and where they struggled the most:
Client-side logic was easier for AI than UI/UX tasks.
Claude 3.5 Sonnet solved 23.9% of Application Logic (Client-Side) tasks, while GPT-4o only managed 8.0%.
For UI/UX work, success rates were even lower, with Claude at 31.7% and GPT-4o at just 2.4%.
AI struggled most with system-wide quality and reliability tasks.
Across all models, performance on these tasks was effectively 0%, meaning AI is still incapable of handling broad, system-wide debugging or QA at a meaningful level.
Bug fixes were where AI performed best.
Claude 3.5 Sonnet solved 28.4% of bug-fixing tasks, outperforming GPT-4o (9.6%) and o1 (19.2%).
However, new feature development remained difficult, with Claude solving only 14.3% of those tasks and GPT-4o failing completely on them.
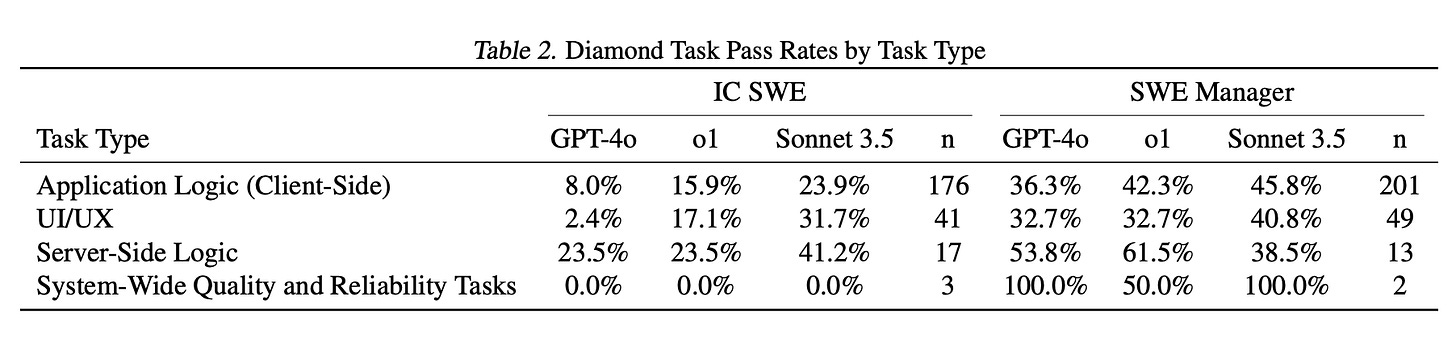
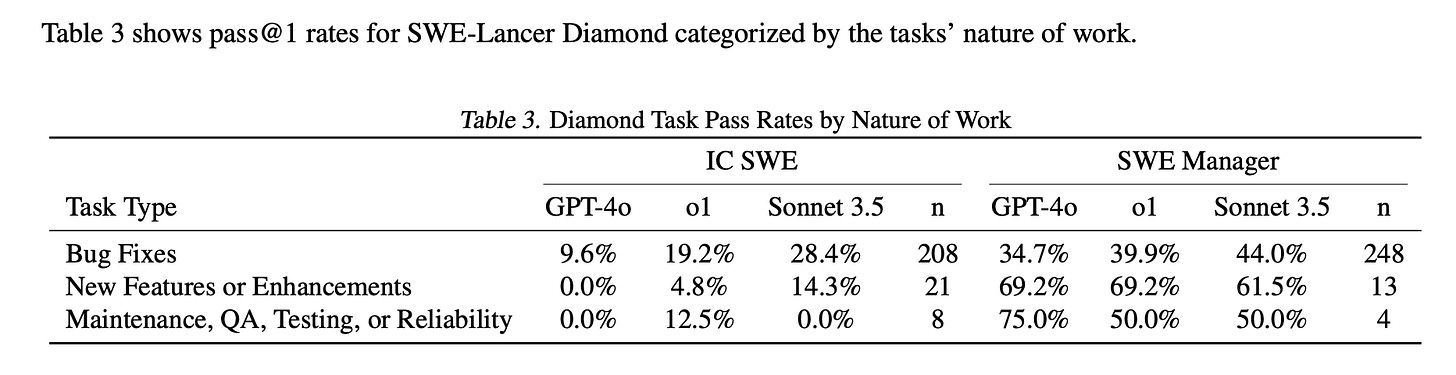
These results suggest that AI models are best suited for narrow, well-defined coding problems like fixing specific bugs or minor application logic errors. However, when it comes to bigger-picture tasks like system-wide reliability, UI/UX improvements, or adding entirely new features, LLMs still fall short.
Why the SWE-Lancer benchmark matters
SWE-Lancer isn't just another AI coding test—it introduces a new way of measuring AI’s impact on the workforce. By mapping AI performance directly to monetary value, it’s like a window into how well AI can compete in real-world job markets.
Here’s why this matters for the future of AI development:
It sets a standard for tracking how future models improve at real-world software engineering tasks.
It highlights areas where AI still struggles, like understanding complex system interactions and debugging real-world issues.
It raises questions about automation in freelance work. If it continues to improve, will AI impact the demand for human freelance engineers? Will it augment human work, or eventually replace some roles?
Wrapping it up
A key takeaway is that AI models struggle much more with hands-on coding than with evaluating existing solutions. This suggests that, while AI can assist in technical decision-making, it still lacks the practical problem-solving skills needed for end-to-end software development.
For now, AI still has a long way to go before it can truly compete with human software developers. But as models improve, SWE-Lancer will help answer an increasingly relevant question: when—if ever—will AI be able to make a living in the gig economy?
Written by Shanice.